Artificial Intelligence has evolved substantially over the last decade, especially in the field of deep learning. Utilizing computer systems to perform tasks that normally require human intelligence has huge promise in applications like visual perception, speech recognition, decision making and language translation. The two main fields in AI are symbolic AI and deep learning. While symbolic AI focuses on representing problems, logic and search with symbolic equations, deep learning focuses on multi-layers of processing in the form of an artificial neural network to extract high level features from data.
As the study of deep learning continues to evolve, the applications utilizing deep learning continue to expand. The advances in pattern recognition, language processing/translation and visual recognition drive the revolution that AI will have on everyday lives. From being able to send a verbal command to a computer, robot or self-driving car, to finding hidden patterns in the chemical and biological impacts of today’s medicines, deep learning is making advances in many fields. One day the rovers on Mars will be controlled by AI algorithms mitigating challenges arising from the communication delay between Earth and Mars.
Advancement in deep learning is largely driven by the ability to process more data and computations leading towards greater performance. However, this increase is limited to the processing power available. In addition, to date deep learning has only successfully tackled system 1 tasks of human cognitive capacity, which is the ability to perceive the world leading to object recognition and language understanding. The research into solving system 2 tasks or the ability to come up with a deliberate sequence of tasks is still in its infancy. Current deep learning techniques are not able to generalize well, based on limited experience which includes extensive labeled data and trials like humans can. Another limitation is that deep learning does not have the robustness required to easily change its environment. Its performance moving from lab to field needs improvement.
Researchers are continuing to use neuroscience to inspire new solutions from the study of neurons to develop soft-attention mechanisms creating frames of reference associations to objects to inferring relevant pieces of knowledge to allow neural networks to better adapt quickly.
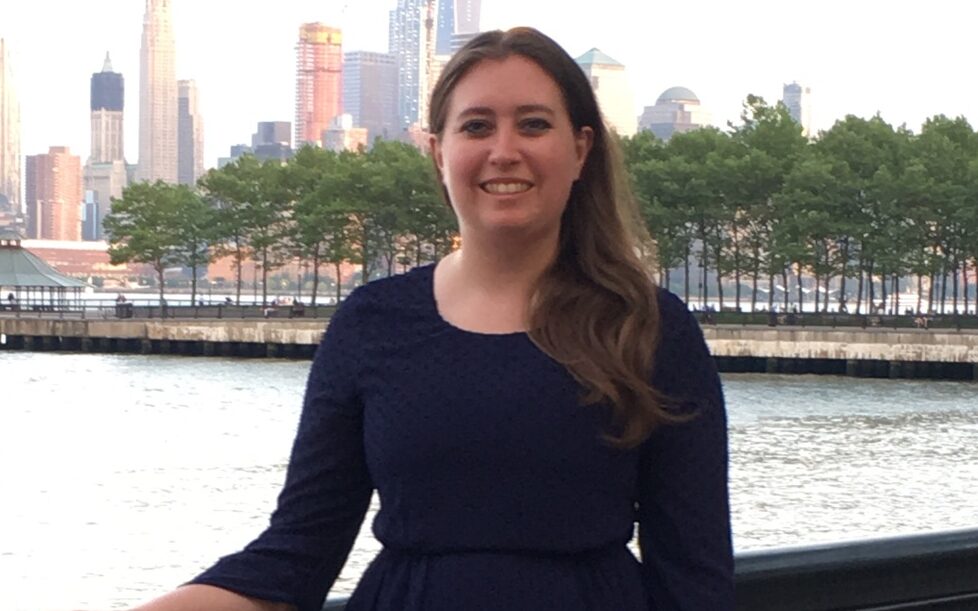
About Lindsey Edwards
With almost 15 years of experience providing data science, automated control system design and software development solutions in the Aerospace Industry, Lindsey Edwards brings cutting-edge analytics to solve today’s business problems.